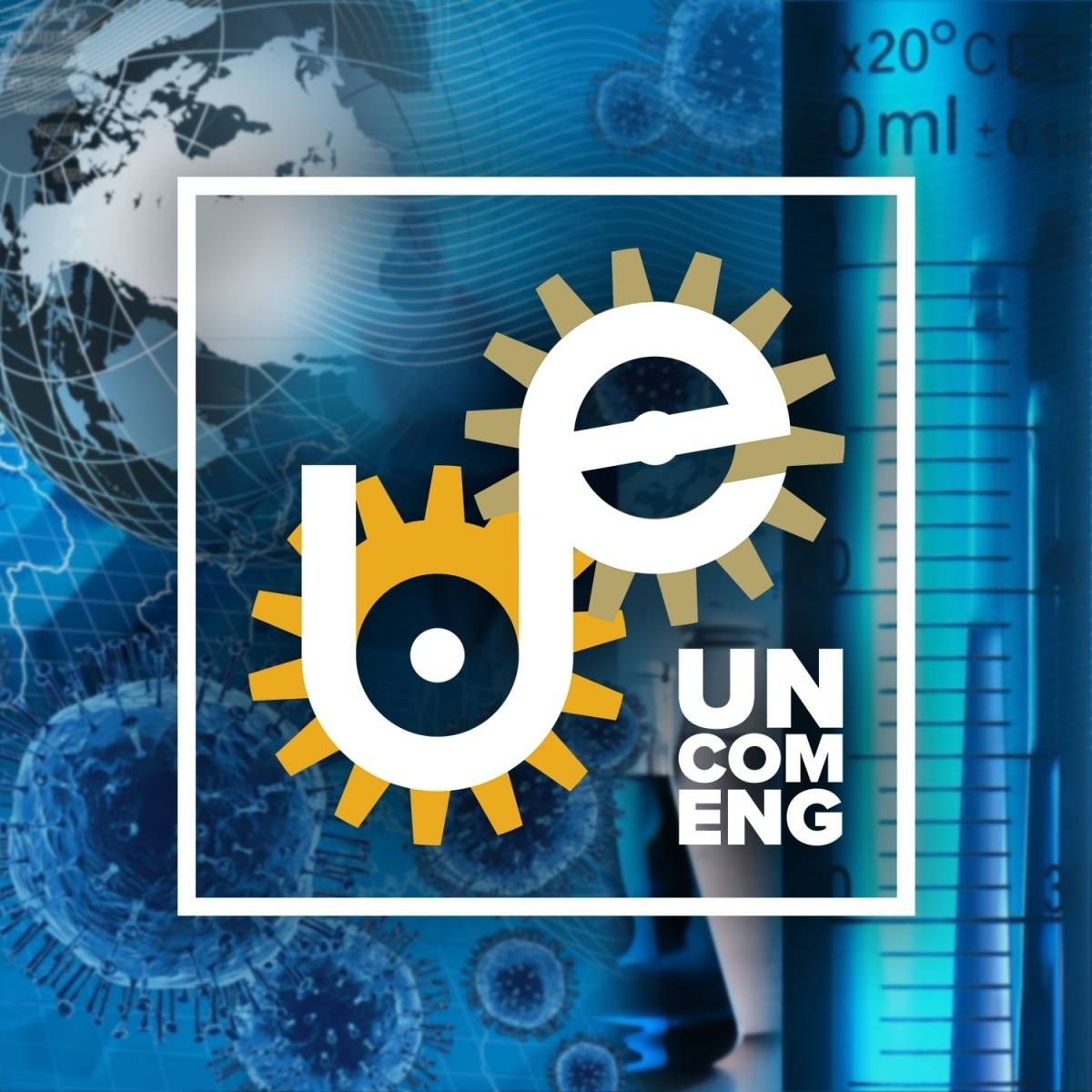
Steve W. McLaughlin
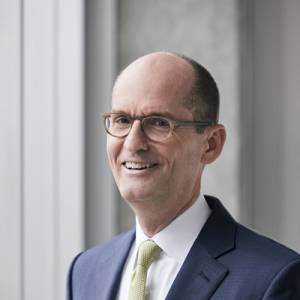
Pinar Keskinocak

Audio
Audio & Captions
Transcript
Steve McLaughlin: In 2020, COVID-19 entered the U.S. and changed our daily lives. Quarantining, face coverings, social distancing, and working from home have become the new normal, and many continue to face even greater challenges. The spread of COVID-19 is flattening out in some countries and areas of the U.S. and still increasing in others, and many experts warn of a second wave in the fall. So how do we move forward in the face of COVID-19 and its continued impact on our lives? And what should we do to plan for the future?
[steam whistle]
[applause]
[marching band music]
I'm Steve McLaughlin, dean of the Georgia Tech College of Engineering, and this is The Uncommon Engineer.
[music]
Man: [archival recording] We’re just absolutely pleased as punch to have you with us. Please say a few words.
[applause]
Steve: Our guest today is Professor Pinar Keskinocak. She's the William W. Chair from the H. Milton Stewart School of Industrial and Systems Engineering. She's also the co-founder and director of the Center for Health and Humanitarian Systems. Welcome to the show, Pinar.
Pinar Keskinocak: Thank you for having me. Great to be here.
Steve: You know, just if it's OK, I just really want to jump straight into COVID-19. You know, we're recording this at a really difficult time for a whole bunch of reasons, and we are going to focus just on COVID-19 right now. You know, we've been working remotely what seems is probably over three months. And I know that your work is really, and is receiving lots and lots of attention around modeling— mathematical modeling— modeling that really predicts where the disease is, where it's headed, who's affected, and how those models get used for planners, for physicians, and so super, super important work. And so I think if it's OK, that's really where I would like to start is for you to share a little bit about the work that you're doing in modeling, and then we can just kind of go from there.
Pinar: Sure. So first of all, thanks again for having me here. It's a pleasure. And let me first start by acknowledging our research team. I am one member of the research team working very closely with my colleagues Nicoleta Serban, John Asplund, and Ph.D. students Arden Baxter, Buse Eylul Oruc, April Yu, Daniel Kim and several others. I'm sure I'm forgetting some names now, but we have a pretty large team and it's been really an amazing group to work with.
So we focus on a couple of different directions. One of the things we try to do is to project the spread of the disease geographically and over time. And we do this by also considering the behavior of people, because how the disease spreads is going to depend in large part, how the public behaves. And so we call these nonmedical interventions, such as school closures, shelter in place. We looked at voluntary quarantine, which means that if a person in a household is experiencing symptoms, not only that person, but all the other members of the household also stay home until the entire household is symptom-free. So these are some of the interventions that we looked at. And in the absence of a vaccine and in the absence of a widespread available antiviral-type treatment, these nonmedical interventions are really our best options at the moment in terms of slowing down the spread of the disease. So that's basically what our disease spread modeling effort focuses on, looking at these different scenarios based on the combinations of interventions that may be in place at any given point in time, and of course, also people's compliance levels. Not everybody is going to comply with the interventions at the same level, so how does the disease spread the change, depending on the combinations of interventions as well as people's compliance levels. So that was one of the research focus areas for us.
And then we also look at the impact on healthcare capacity, specifically looking at the demand for hospital beds, ICU beds, and ventilators, so under these different scenarios, what kind of demand do we see specifically for COVID patients for these resources and also try to project where we might see shortages and when and at what levels.
And then finally, we also look at hotspots, looking at the trends and the changes, specifically in the data in terms of confirmed cases— can we say that certain areas are likely to become hotspots in the coming days, which gives us opportunities to better prepare for response in those areas. So those are some of the focus areas of our research team.
Steve: You know, there's so many aspects to being able to accurately predict, you know, what's happening. And you touched on, you know, your medical aspects. You touched on human behavior aspects. You touched on all kinds of various things. And I know many of our listeners are high school students, junior high students, engineers, engineering graduates, so I know, certainly I am, I think a lot of people are interested in how do you develop such a model? You know, what does a model look like and what are the kinds of tools? And how do you incorporate all this really varied information into something? Because, I mean, the other question is you're an industrial engineer, and, you know, I think people don't necessarily think of engineers as developing a disease spread model, so I know I just threw a bunch of things that you there, but people are really, really curious about the model and how you develop the model and do that.
Pinar: So maybe I'll just give a very quick overview of the different types of models, because there are so many models out there, and broadly speaking, they fall into one of three categories. So we have what I would call the “curve-fitting models”. These models look at the data, the spread data [of] confirmed cases. And then, just like you will do, let's say in a forecasting model, they try to look at the trends and then from there try to project how things might look like, assuming more or less if similar trends follow. So these curve-fitting models tend to be quite good if you are looking forward, let's say, one or two weeks, maybe up to three. But beyond that, it's difficult for them to capture some of these other dynamics that we discussed earlier, such as human behavior or different interventions and so on. So that's one category.
And another category is what we call “SIR models”, standing for susceptible, infected, recovered. But these models basically put people into one of three categories. Because this is a pandemic, initially none of us have any immunity for the disease, so we are all susceptible. We all could get sick because we don't have any prior immunity in our body. And then at some point in time, a person may get infected and then eventually, you know, hopefully they recover. So you fall into one of these three categories, each individual at a point in time.
And then the recovered category basically assumes that once you recover, typically you will not get infected again. So we don't know if that's the case exactly for COVID yet, you know, hopefully or most likely that is the case. So these models can track these some of these different interventions to some degree, because we can say, OK, we have a school closures, then that means some of the interactions are going to be lower, the transmission is going to be lower. So they are able to incorporate some of these to some degree, but not usually at the high, high level at the population level, maybe at the subpopulations divided into age groups.
And then the third category is what we call “agent-based models.” That's the type of model that our team developed. And in the agent-based models, you have an agent and entity in a simulation representing each individual in the population. So we are essentially mimicking the entire population in a simulation model. So each of these individuals, what happens when you get sick? Some people might experience symptoms. Other people may not experience any symptoms. And then depending on whether you are symptomatic or asymptomatic, there's a chance you might pass the disease to other people. So there is kind of the individual, the progression of the disease within an individual, we capture this depending on age, and it's not the same for everyone. And then we also capture the interactions in the population where we model interactions in a household, interactions in a peer group which may be school or workplace, and interactions in a community such as a grocery store or a church or other public places that people get together. The agent-based models, in a way, capture a lot of the details that happen in the population, and they also make it very easy for us to implement and test different intervention strategies such as the ones that we discussed—school closures and volunteer quarantine and other disease— other interventions.
And the nice thing about the agent-based models is that even if an intervention has not been tried before— so we may not have data to fit for that particular type of intervention— we can still incorporate it in the model because we are explicitly modeling the specific human behavior. So they have a lot of flexibility. They are more complex than some of these other models and hence they take a bit longer to run, but they give us a lot of flexibility to do different things. So I mean, there may be other types of models, but broadly speaking, most of the models we see out there would fit into one of these three categories. And I think as a researcher, it's fantastic and wonderful to see these different types of models implemented and used by different research groups, because I think we learn something from each of them. And when we look at the results, you know, in an ensemble incorporating the projections from these different models, it gives us a lot of, you know, opportunities to observe what may happen in the in the best case, worst case, average case. And so I think it's nice to have these different options.
The CDC has on their website actually a collection of these models in ensemble, our model is also, you know, one of the models in the ensemble. And I would encourage everyone who is listening to take a look at it and see how these different models come up with different projections over time.
Steve: It feels to me like it exists at several scales, right? It sounds like you're able to model an individual and that individual's interactions with different groups within family. And then there's another scale around how those different groups might interact or how the disease spreads beyond those groups, and then at an even greater macro scale, how it transitions across states and country boundaries and things like that. Is that is that true? I mean, your model, even just the agent-based?
Pinar: That's exactly how it works. And so we assign each agent, each person, in our simulation to a household so they have a household. And then we use data specifically, you know, our model is focusing in terms of data on Georgia. We use Georgia data in calibrating the model, in populating the model, but it could be applied to any other geographic region, really. But we would use, for example, depending on your location, on the agent's location, what would be the household size. So all of that information we get from the census. So each person in the simulation belongs to the household, and then they belong to a peer group depending on their age— this may be a school group or a work group. Again, we look at the data in terms of school sizes, depending on the location, the school size or the work group size might differ. And then people travel. They travel for work quite a bit. We have the work travel data from Georgia. So you may be living in one county in one census this track, but traveling somewhere else, so then this is going to create interactions between different locations because people from different locations aggregate in a workplace and then go back home. And then as we discussed earlier, we also have the community interactions that, you know, you may be getting together with people from all over the state in some cases, probably more so from your own community.
And then in terms of transmission, the chances of transmission, you might imagine, is higher if you are in the same household with someone. It's a little bit lower if you are in the same workplace or peer group such as school. And it's even lower if you are interacting in the community because you are not spending so much time with them. So all of these different effects of transmission are incorporated into the model so that we can capture, you know, the likelihood of transmission depending on the interactions.
Steve: I mean, it just seems so incredibly complex, right? How you both build the underlying mathematics that describes all these things, but then more importantly, you want to be— the model needs to be informed and needs to have the right data. And whether the community— I don't know who I mean by “community”— but whether the community cooperates in providing that data, whether the community competes to share data, how easy is it for you to kind of pull those parameters broadly into your model? And I guess the last thing I'll say, I can now understand why your team is so big, right? That you know—
Pinar: Yeah. So I mean, the data part can become really tricky. So thankfully, a lot of the data comes from the census. So in terms of the, you know, age distribution based on location, the household sizes and so on and so forth, as well as the group travel, so that data is available. And, you know, we, of course, have to do quite a bit of work to take that available data, clean it, and then put it into the model, but it is out there.
What is more complicated is the behavior-related data. How do you know what percentage of the population will actually comply with a shelter in place order? Or after shelter in place ends, anecdotally we know that some people are pretty much continuing voluntarily shelter in place, staying working from home and not interacting with others, whereas other people are not. So how do we know, you know, exactly what these percentages are? Of course, we don't know, so we use some estimates. For example, there is data available based on cell phone usage showing over time how far people travel and for how long, so that gives us some idea about the changes in behavior patterns, you know, how it was when you were in a shelter in place and how it's changing now over time. So we basically look at that kind of data and then that informs our model in terms of developing some scenarios. For example, we might say, let's say 80 percent of the people were following shelter in place, and then we fit the model and compared it to the actual data and see if that gives us a reasonable estimate. And then we might say, what if maybe 60 percent of the people continue sheltering in place one week after? [It] maybe drops to 50 percent two weeks after shelter in place ends, but they are still voluntarily continuing. But will it this tapers off and then we reach maybe a plateau? Maybe five percent of the households are still continuing, and everybody else is going back to business as usual. But we can test a little of these different scenarios. And then as new data becomes available, we compare and contrast the model predictions with what we see in the data based on the actual confirmed cases and then make adjustments if needed. But because we are able to test a lot of different scenarios, for the most part we are able to capture what the range might be, you know, depending on the different behaviors and how it reflects in the actual number of infections we see.
The one thing that makes it really, really challenging is the fact that our testing capacity has been quite limited, which means that there may be many more infected people as well as infected and recovered people out there that we do not know about. So therefore, what we do is we take the reported numbers and, again, based on estimates, we say maybe the actual numbers might be about six or seven times what the confirmed cases are based on the testing availability and other information that we have. So there is a lot of— there are a lot of estimates in both calibrating the model parameters as well as how we interpret the number of confirmed cases that are out there.
So I guess despite the uncertainties, I think the models still give us very good information about what might happen under these different scenarios, under these different interventions, because otherwise we will be literally flying in the dark, you know, not even knowing the impact of, you know, a particular intervention or relaxing an intervention and, you know what we might see. And these are the kinds of situations where doing an actual experiment— oh, let's try and see what happens socially, economically, and from a public health perspective, very expensive experiments, right. I'm using the word “expensive” in a very general way, so I think that's where the models are quite helpful, you know, in terms of helping the decision makers to make more informed decisions.
Steve: As most are familiar with, you know, hurricane models, right, we see all these pictures of, you know, the eight or 10 models that show the pathways of the hurricanes and so on, and I keep— one, I don't I don't want to oversimplify it and I don't want to offend, you know, you or others, you know, kind of trying, if there is or is not too much equivalence, but then, you know, when you talked about the models that the CDC has posted, I just have a whole bunch of different questions and thoughts. And I don't want to, like, stray into politics at all because it seems like that there's a wealth of— there's a wealth of models. Like you said, there's an ensemble of models that probably point to some concrete conclusions. And I'm curious about, you know, number one, how well the modelers, the different— I don’t know how many different models there are out there— that would be interesting to hear, you know, and then as someone assembles, you know, the general conclusions from those models that those feed into certain kinds of decision-making. And I'm really curious about— because it sounds like you're right in the middle of that— and I'm just curious about like what happens next when you have 20 or 30 or 50 or 100 models and how all that gets pulled together and who uses them, and do you collaborate? Do people work well together on those?
Pinar: And so you are asking a fantastic question— How do we make sense out of these multiple models, and how do we incorporate them into our decision-making? So the models that I was referring to earlier, similar to our model, focus on projecting the disease spread, especially under different scenarios regarding intervention strategies. So in a way, our efforts, as well as some of the other efforts, take the perspective of public health. So we look at a number of infections, a number of infections with symptoms, number of hospitalizations, how many ICU beds might be needed. And we look at it over time so, of course, the cumulative numbers matter— how many people, will be infected through the course of the disease— but we also look at what might happen at the peak, which is the day or, you know, maybe a couple of days a week where this is really the most intense— we see the most number of infections, new infections, daily. So that's what we call the peak. And why does the peak matter? Because that's when our resources will be most stretched, right. So you have so many new infections, so many people who might need hospitalization or ventilators, and that's the resources are really, really stretched at that point, particularly from a public health perspective. So that's why over time we kept hearing this phrase, “flattening the curve,” right. So what is the curve? It starts slow with a small number of infections at the beginning, and then it picks up speed, and then it reaches a peak, and then after that, it will come down. This is kind of the most traditional way. If you did nothing, that's the kind of curve that you would see. But because we are doing interventions, we might actually see multiple peaks. So we are already observing, if you look at the data for Georgia on the Georgia Department of Public Health website, we already had one peak, and then because things slowed down during sheltered in place, we start to see things coming down, and then the number of new patients started to pick up again after shelter in place was released— another peak, right? So there may be multiple peaks because of these different kinds of public health interventions, businesses reopening, and so on.
And so, again, the focus of our model and several of the other models that project disease spread is on public health. So we basically look at what combination of interventions would reduce the number of infections or reduce the peak or delay the peak. So those are the kinds of things we are interested in just focusing on the on the health side. And of course, if you are a decision maker, that's not the only thing you would be considering. So we might find out that if you throw in five or six different interactions or just simply tell people all of you are going to stay home for two months, no going out, nothing; we’ll stop the whole country, yes, that might stop the disease, but that may not be feasible for various reasons. So there are all these other factors that come into play. The closing schools re-quantify public health impact of closing the schools, but then what about the social impact? There are already studies that show that being away from school and having to do schooling online disproportionately affects African-American and Hispanic communities and may increase the gap, and it may be more difficult for certain subpopulations to catch up with school if and when we go back to regular schooling. So those are some of these other considerations. Of course, there is the huge concern about the economy and so on. So I think the decisions are very complex. There are different models that capture different aspects of these decisions. Our model focuses on the public health aspects. There are other models that look at the impact on education, the impact on the economy, and then it's up to the decision makers how to pull the information from these different models, understand to the extent possible the tradeoffs, and then make, hopefully, informed decisions. I wish there was one big mega model that put all of these together and gave us the magic answer about what to do and when, but we are not there yet— maybe in the future.
Steve: So, you know, the modeling work that you do obviously applies to the U.S. and we keep hearing various hotspots around the world. I assume your models are just as valid, you know, around the world, and you've traveled all around the world for this kind of work and others. And so I'm really curious about your perspective on what you see happening either right now or in the coming months in other parts of the world.
Pinar: Yeah, I mean, needless to say, I am very concerned about the impact of COVID in countries where the population density is high. The infrastructure, whether it's health, infrastructure or water, sanitation, hygiene infrastructure, may not be anywhere near what we have in the United States. And some of these measures that we recommend— physical distancing, frequent hand washing, and so on may just not be feasible to the extent that they are here. So given what we see in the United States, considering the development that we have here and thinking about what might happen in other countries where they do not have the kind of infrastructure that we have, it's very, very concerning.
So the other thing that's of big concern to me is the fact that a lot of progress happened around the world in terms of childhood vaccinations and establishing a basic health infrastructure for primary care, and now what we see is a lot of funding and efforts that were primarily dedicated to those areas are now shifting because of necessity to COVID. And I think there's a huge concern that the progress that we made in childhood vaccinations, for example, might slow down or even take a back step because we might not have the funds and resources to continue some of those efforts as we also struggle with COVID under very, very limited resources. So when I think about the developing world, my concern is not only for COVID, which is a huge concern, but also about some of these other areas— basic health care and vaccinations and so on. I think a lot of those areas might also get a hard hit. And then clearly the education side, you know, school closures have been difficult for certain communities in the United States, continuing education online and, you know, I can only imagine how difficult this will be in some other countries around the world. So, yeah, there are I mean, I'm sorry for sounding quite grim, but there are lots of concerns.
Steve: Well, you know, Pinar, you're a member of the Georgia Tech community. You're going to be presumably teaching in the fall advising your students. I know we're all working remotely, you know, figuring out some way to get back to campus, and I'm really curious both in your modeling experience, you know, what your view on the fall or what the models are predicting or not, and what you think the universities are facing for the fall, and you're right in the middle of all that.
Pinar: I think when you bring a large group of people in a relatively small space such as campus, the chances of the spread happening certainly goes up, right. So you don't even need the model for us to say that just because of the proximity and the duration of interactions in a relatively small area. Having said that, what happens is going to depend to a very large extent on how our faculty, students, and staff behave when we get back to campus. There are several physical distancing measures that are recommended in terms of, you know, we need to stay six feet apart whenever possible, wear a mask, wash hands, and so on and so forth— so both hygiene and physical distancing measures. It is very difficult for me to say what percentage of our campus population, depending on who they are and where they are, will follow these. So I think if the compliance levels are quite high, then we might be fine, and we might actually not see a huge surge in any way in infections. If the compliance levels are low, we may be facing some challenges. So I guess that's why I have been really promoting doing some sort of survey, and not just a one-time, but kind of recurrent, to get a better feel about what people's current perceptions and attitudes might be, and then depending on that, how we communicate the impact of different types of behavior. So, I mean, I know I'm not answering the question directly, but I think, again, to a large extent, it will depend on how we behave.
Steve: Well, I suspect that your work on COVID and modeling is an outgrowth of things that you've been working on your entire career. And I know that you are one of the founders and now the director of the Health and Humanitarian Systems Center here at Georgia Tech, and I really would love to hear more about what that center does, the kinds of work that you and your colleagues do to try to bring these same kind of models to different settings. I'd love to hear more about it.
Pinar: Oh, I would love to talk about that. So we started the center in 2007 together with my colleagues Julie Swann and Ozlem Ergun. Unfortunately, both of them left Georgia Tech now, but we still work very closely together with them. So we had our research in logistics and supply chain management, and all of us had done work in that space for some time, worked with the private sector, had impact. We had seen the impact of our work in the private sector and that was really, really satisfying. But we were all asking ourselves the question— So it's great to help companies become more efficient and more profitable, serve their customers better, but how do we take this knowledge and expertise in our research to the public sector so that we can more directly impact human life, people, directly? So that motivated us to get started. And initially, the Center focused primarily on the humanitarian space, both disaster preparedness, response, recovery, but also long term development especially focusing on the on the developing countries or other areas. But then we quickly saw that a lot of the work that you are doing was expanding into health space. And in some cases, we were seeing a lot of overlap between health systems and humanitarian systems. You know, and we've seen this in some of these recent disasters. For example, you may have a natural disaster then followed by a cholera outbreak. Or in case of the Ebola outbreak, we've seen how a lot of these systems really had to work together. So that's how the scope of the of the center quickly expanded from humanitarian space to health space. And I would say right now, probably more than 50 percent of what we do is specifically focused on focused on the health space and still quite active on the humanitarian space.
In terms of the disease modeling, one of the first projects that we had done was actually on pandemic flu, H1N1. Some of our listeners may remember H1N1. And we started collaborating with the Atlanta Red Cross and they were put in charge of— actually, this was even before H1N1— they were put in charge of planning for food distribution in case of a pandemic. And they approached us and said, “Well, we need to develop a plan, but we do not know how many people we may need to feed, why those people might be, and how such a plan might look like.” So that was the first time that we started developing a model to project the spread of the of the disease. And then we looked at different scenarios, just like we are doing now. in terms of intervention strategies and what if we feed only people who are below a certain income level and so on and so forth, and then we worked with them in the development of such a plan. And around that time, we also got in touch with Georgia Department of Public Health and Georgia Department of Education. The Department of Education was interested in school-closing in case of a pandemic. Would it be able to close schools? What's the impact, and so on? So it's very much similar to what is going on right now. And we actually used the flu pandemic model that we had developed then and expanded it and adapted it to the pandemic situation today. So that work certainly came in very handy.
And then, actually that was a very nice opportunity for us to see the amazing connections between disease modeling, public health, and industrial and systems engineering, supply chain management in particular, because on the epidemiology side, you look at the projections, disease projections, and that industrial engineering supply chain management focuses on how do we allocate resources so that we can actually take care of the demand, right, so how do we better match the demand and supply. In our work at the time by combining this infectious disease modeling with optimization methodology to see how best to do this food distribution really brought these two different fields together in a very synergistic manner. And that's been one of the focus areas of the Center over the years, bringing together expertise, ideas, modeling approaches from different fields so that we can actually tackle some of these complex problems.
Steve: So one of the things that we often talk about here on The Uncommon Engineer is your own path to finding your way to becoming an engineer and to becoming, you know, a faculty member. So I'm really curious about, you know, when you were younger, what are the things that happened or that you experience that brought you to a career, a fabulous career, in engineering.
Pinar: Yeah, I guess the main reason I became an engineer is because I love math. So that that has been my favorite topic all through my school years. And I could study math, but the career options at the time in the country that I grew up in Turkey were quite limited if I had studied math. So then the next option was engineering from the perspective of doing math and yet having more opportunities and flexibility in terms of career options. So that led me into engineering. And in terms of the area, specific area within engineering, the three options at that time were electrical engineering, computer science/engineering, and industrial engineering. And I kind of looked at the descriptions. I didn't know any of them because I didn't have anybody in my family who studied in any of those fields before. I didn't know anyone who studied in those fields before. It was mostly based on reading and trying to figure out, you know, what am I going to do after I graduate? And industrial engineering seemed to have the most flexibility in terms of applying what you do with math and also combining it with human impact. So that's basically what led to my choice for industrial engineering. So in those days, the opportunities for collecting information weren’t nearly as much as what we have today, so this was very limited. But it turned out to be a logical choice. I never regretted it and really enjoyed, you know, studying industrial engineering and then becoming a professor eventually. So if it was up to my family, engineering was probably wouldn't be their top choice for various reasons. My mom would have loved it if I became a doctor, but I knew that I wouldn't be able to survive the medical school. I would probably faint at the first sight of blood or some other thing, so I said that is not going to work. But it's kind of amazing how I managed to find a path at the intersection of engineering and medicine and health systems, eventually honoring my mother's wishes, even though I didn't become a doctor, managed to find a way to bring the two sides together. So that's been both professionally and personally really, really wonderful.
Steve: It's really— I resonate really well with that because I love math, too, chose electrical engineering to do math. I think for students out there that— because so many students, junior high, high school students, don't know what engineering is, and I always try to say, because most of those students, you know, the high school guidance counselor tends to say, if you're good in math and science, you should study engineering, right. And I think even for those students who really, truly love math or who really, truly love physics but do want to make a difference in people and connect to people, that engineering is a great choice. And everything that you said for the last hour is just a perfect example of that. At your heart and soul, a mathematician, but you're having obviously a huge impact on people.
Pinar: To an audience that was not engineers, this was a very broad audience from all ages. And I started by my talk by asking them, just think about the words “math” and “engineering” and just think about what kind of images those words bring to you, and just take a moment. And I again, would like our audience to think about these words, “math” and “engineering.” And most people wouldn't think about making the world a better place and saving lives and improving our health systems. Not necessarily those images come to mind, but that's what I want a lot of people to think about. When they think about math and engineering, I hope more people think about making the world a better place. So I think that's what a lot of engineers do on a day-to-day basis. It may not be that obvious— the connection— but the engineering education gives such an amazing foundation that you could really do pretty much whatever you want to do with the foundation, whether it's in health systems or some other area where you would like to have impact. So hopefully more and more young people, as they think about career choices, think about engineering in that way.
Steve: I couldn't agree more. I think, yeah, for young people who want to make that kind of a difference, they'll find a way to do that.
I can't thank you enough for spending time with us. You’ve just had an absolutely outstanding career at Georgia Tech. We're so lucky to have you here. The impact that you're making on our students in the classroom, but more importantly, the impact you're making to all society is just a perfect example of what can happen. So, Pinar, thank you so much for coming on, and we're really going to be on the lookout for all the fantastic work that you're going to keep doing. So thank thanks so much, Pinar.
Pinar: Thank you so much for having me. I love Georgia Tech. I’ve loved, you know, being here for so many years. It's a fantastic environment. I love my students. And the collaboration with colleagues is amazing. I think that's one of the unique aspects of Georgia Tech— we have such an amazing collaborative culture here and working together with others. And this may be one of the other things that I would like people to think about— being an engineer doesn't mean you are going to sit in a room by yourself and work on something in isolation; it's a very, very collaborative work environment, and it's so much fun to solve these puzzling problems and attacking the challenging issues together with others. So thanks again for having me.
[marching band music]
Geekout
Audio & Captions
Transcript
[scanning radio dial]
[big band swing music]
Steven McLaughlin: —sounds incredibly complex—it sounds like—you need to have abilities that span—I’m really geekin’ out here.
[laughter and applause]
[big band swing music]
Steve McLaughlin: I'm curious about one of the other humanitarian crises or events that you've been involved with or help model. Is it a tsunami? Is there earthquakes? Is it all of the above? I'd love to hear more about a specific example that kind of also led into the work that you do now.
Pinar Keskinocak: Yeah, so maybe because we live in the southeastern part of the United States, and this is one of those areas— it's not if we will have a hurricane, but when and where we might have a hurricane and the impact. Some of our work focused quite a bit on hurricanes, not so much on predicting the path of the hurricanes; so that's our civil and environmental engineering and other colleagues that do a fantastic job of there. But we focus more on preparedness and also response, so let me talk a little bit about each.
So let me first kind of go to the response side. So when a natural disaster happens, whether it's a hurricane or an earthquake, typically there is a lot of debris on the ground. This could be tree debris, you know, buildings. Depending on the type of disaster, you might see different types of debris. This topic may not sound that exciting, but it has a huge impact on response and recovery efforts. So when there is debris on the ground, you cannot reach people for search and rescue operations. It becomes very difficult for people to evacuate the area. It becomes very difficult for us to bring in supplies and personnel to help. It becomes difficult to restore the infrastructure. For example, if you have a power outage, you can only go and fix the outage in a particular area if you can actually access that area. So that is one of the things, as an example, that we focused on. If we have an area that some of the roads are at least partially blocked by debris, what is a good plan to clear the debris so that we can actually restore life to normal or deliver goods and services or get people out in the most efficient and effective manner? So that's one of the areas that we have done quite a lot of work on.
Another area, which is more on the preparedness side, is on inventory pre-positioning. We have done several projects that are, as one example, I'll talk about a project that we have done with CARE. Again, as some of our listeners might know, is a large nonprofit organization. It’s headquartered in Atlanta, but they do most of their work in in other countries. They operate in over 90 countries right now, if I'm not mistaken. And they respond to disasters as well as doing some work in the development space. So when you first engage with them— and this was true for many of the other nonprofit organizations— their procurement for disaster response would happen, for the most part, after a disaster. So something happens, and then they see the need, and then funding donations start to come in, and then they would go to procurement and then try to deliver the supplies. Of course, as you can imagine, this creates issues in so many different ways. Timing is a huge concern. By the time you are able to negotiate the contracts, do the procurement, and then actually deliver the goods there, that the clock is ticking. The first 24 to 72 hours after a disaster are crucial in terms of saving lives in either helping people or getting them out. And this approach would make it very difficult in terms of a timely response. And from an economic perspective, also it poses challenges, because after a disaster, a lot of organizations are going after the same suppliers, same transportation providers, so sometimes you will see that the cost of a good would go up three, four, or five times the normal, you know, the normal price. So we looked at pre-positioning supplies for disaster response, considering different scenarios and looking at historical data of what happens in certain regions. We basically looked at worldwide where would we pre-position these supplies, reach supplies in what quantities? And then we looked at the tradeoffs of pre-positioning versus shipping after the fact, both on timeliness and cost. So those are some of the some of the projects that we have done over the years on the disasters space, both in the preparedness side and also on the response side.
Steve: And, you know, as you were talking, again, I don't think I'll make too much of a political statement here, but, you know, this idea that— and it's often difficult for decision makers to do that advanced planning because to spend money, to spend resources now for something, and in many cases, you know are going to happen. In this case, it was something like people had really hoped was never going to happen.
Pinar: Mm-hmm.
Steve: I guess my question— so I understand that, and it's really fascinating that, you know, pre-positioning and doing all this stuff ahead, it's probably just a pretty simple question— How much does it save by doing that kind of pre-positioning? I mean, are we talking about— it's obviously a huge savings in time, but even just dollar amounts, you know, a dollar that I invest now today saves two dollars? Ten dollars? A hundred dollars? What's a typical kind of a thing?
Pinar: Significantly. I think all of the numbers do you put in, depending on the scenario, could be realized. For every dollar we invest, we save $3, $5, $7, or even $10, right. So depending on the scenario, I think sometimes the difficulty is that you spend, you invest in prevention, and then because of the investment you made, you don't see a huge, you know, impact. And then some people might come and question, “Why did we spend all that money and nothing happened?” Right? So I think that's the hard part to quantify with prevention. So that's one piece.
The other piece is that when there's a disaster, it's easier to motivate people, policymakers, to spend the funds to stop it or to mitigate the negative impact. But before that happens, it's not that easy to make the case. So, you know, they might think, “Well, maybe they're just crying wolf. There is really no danger here. Let's wait and see if something actually is going to happen.” I mean, I think an excellent example is evacuation, right? So how do you make a hurricane evacuation decision? Because if you if you evacuate people and then eventually maybe the event is not as big as you predicted, you under fire. Why did you vacate people? What a waste of time and money. If you don't evacuate them and then something really big happens, now you actually may have cost lives. So these are very difficult decisions because we are making them under huge uncertainty, and we need to weigh the pros and cons of doing something versus not doing something. And again, if all goes well, you might be criticized for, you know, why did you do this and waste money. But the cost of not doing it might sometimes be much, much, much, much higher.
Steve: And as an outsider or, dare I say taxpayer, that's why those models are so important, right, that the more accurate, the more detail, the more specific that those models can be and the more complex they can be, I don’t know how easier those decisions are, but the more informed those decisions are.
Pinar: Right. Right.
[marching band music]